Would you trust an AI Operative in the field?
A world-leading expert in artificial intelligence (AI) questions the rosy views that dominate thinking in the development of AI technology. As Program Lead for Artificial Intelligence at the University of Melbourne, Professor James Bailey says the conversation neglects important nuances regarding the technology’s underlying fragility.
People don’t necessarily realise how brittle a lot of AI actually is,
he says. AI systems tend to respond well to what they’ve been trained to detect, but responses can become erratic when confronted with novel or unexpected circumstances.
Examples of this brittleness include autonomous vehicles whose AI could not correctly navigate when confronted with snow on a road – a seasonal phenomenon not experienced during training in warmer locations like California.
The wider issue is that currently there is no standardised solution to the problem of how to verify an AI system,
Professor Bailey explains. This is as much a research problem as an industry need, with advances essential for the technology’s wider adoption.
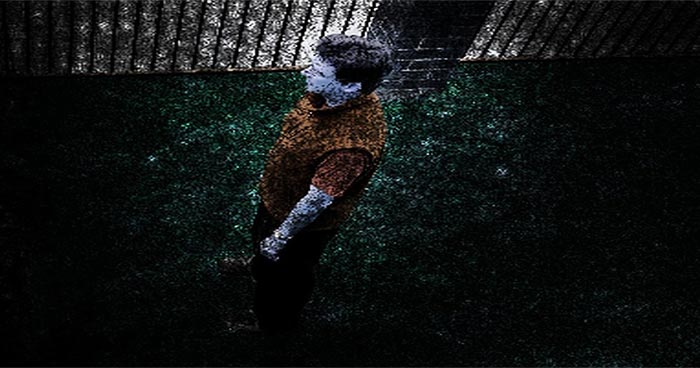
In 2019, the University of Melbourne launched the AI Assurance Lab to work on this challenge. The goal is to dissect, analyse and solve AI’s inherent fragility in order to better meet the needs of industry, defence and governments.
AI systems tend to respond well to what they’ve been trained to detect, but responses can become erratic when confronted with novel or unexpected circumstances
Interested in learning more about AI? Learn more about the Master of Information Technology
The issue is far from trivial
Weaknesses in an AI system are exceptionally difficult to detect and resolve. They cannot be spotted as lines of problematic code. It may also be impossible to train an AI before deployment for all the contingencies it is likely to encounter. Most importantly, current AI systems are unaware when they are making a potentially disastrous mistake.
To make headway, the AI Assurance Lab has tackled the problem by first breaking down what ‘assurance’ means in the context of an AI system.
Our approach means we can identify sub-traits that correspond to important facets of the greater verification problem,
Professor Bailey says. These sub-traits then make it possible to develop refinements that make targeted improvement possible.
One assurance sub-trait that is easier to understand is an AI’s ‘resilience’, which refers to its operational integrity in unexpected circumstances or in variable conditions. This is the trait that misfired in the self-driving vehicle that was stumped by snow. ‘Resilience’ has to be addressed up front by development approaches that ensure relevant scenarios are included when training an AI.
Another trait is ‘explainability’, which involves providing an AI system with the ability to explain why it arrived at a decision. An example of this is an AI ‘explaining’ why it classified the image of a structure or object as a building or a particular person.
Many AI systems are currently not very good at explaining their decisions and that’s a problem where humans and AIs work together because it gets in the way of building trust,
Professor Bailey says. Explainability is essential to building trusted partnerships.
This trait is particularly important in the defence sector. Professor Bailey points to the deployment of a robot within a Marine Corps unit that was trained to perform scouting and navigation duties. Trustworthiness – in this case, the robot’s information – is essential.
Related to this is the trait of ‘competence’, the ability of AI to recognise limitations to its own competence when processing an input it has never ‘seen’ before. Professor Bailey describes this as a very active and very difficult area of research.
The impacts can be profound. We have examples of a T-shirt design that prevents AI facial recognition software from recognising that image as a person
Then there are traits related to situations that involve malicious intent. This includes ‘adversarial machine learning’, which involves situations where external actors deliberately attempt to fool an AI by exploiting weaknesses in its resilience and competence.
Professor Bailey provides the example of cleverly designed stickers placed on a road that tricked self-driving Tesla cars to change lanes unexpectedly. In another example, a stop sign became invisible to an AI vision system by the addition of a specific pattern of rust, while still remaining visible to humans.
We are seeing that people can deliberately exploit the brittleness of AI systems using clever but simple changes to the input,
Professor Bailey says. The impacts can be profound. We have examples of a T-shirt design that prevents AI facial recognition software from recognising that image as a person.
Another adversarial trait involves ‘back doors’ in which malicious software is hidden in an AI system and behaves like a sleeper agent. The AI behaves normally until it encounters a pre-programmed trigger, which can include an object in an image or a particular audio frequency. The system will then do something totally unexpected. These issues are particularly pertinent for open source AI technology downloaded from the web.
The effects on the AI’s performance depend on how it is set up,
explains Professor Bailey. For example, a particular sound frequency might be used to fool a speech recognition system at a call centre. Unfortunately, there is currently no easy way to detect back door sleeper code.
It is traits like these that are seeing the AI Assurance Lab work on AI verification to deal with issues facing industry and defence when it comes to choosing an AI system to suit their needs or when an organisation develops its own from scratch.
What is already apparent to Professor Bailey is that adopters of AI technology will face some stark trade-offs to ensure their system is trustworthy. For instance, one way to defend against adversarial attacks is to make the AI system very simple. That makes the AI hard to fool. The trade-off is that simple AI systems are less accurate, while complex AI systems are more accurate but less robust.
That’s the challenge in this kind of research,
Professor Bailey says. How do you trade off equally valuable traits, such as robustness and accuracy, because it appears to be very difficult to get both at once.
Getting the balance right is going to prove critical for the adoption of AI technologies. At stake is AI’s enormous potential for gains in automation and in its ability to process data at far greater scale, speed and accuracy than humans.
For more information visit the AI Assurance Lab.